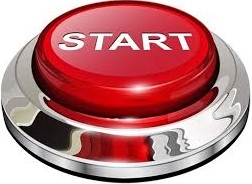
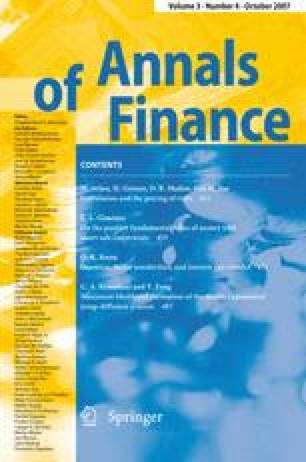
In Section 4, an overview of the data set is provided, and then three procedures in the predictive classification problem are introduced. The method of proof consists of reducing the original optimal stopping problem to a free-boundary problem. In Section 3, we provide a general jump-size detection analysis based on the sequential testing of hypotheses. Several examples are presented.ĪB - We study the Bayesian problem of sequential testing of two simple hypotheses about the Lévy-Khintchine triplet of a Lévy process, having diffusion component, represented by a Brownian motion with drift, and jump component of finite variation. We show it is characterized by a second order integro-differential equation, that the unknown value function solves on the continuation region, and by the smooth fit principle, which holds at the unknown boundary points. The method of proof consists of reducing the original optimal stopping problem to a free-boundary problem. N2 - We study the Bayesian problem of sequential testing of two simple hypotheses about the Lévy-Khintchine triplet of a Lévy process, having diffusion component, represented by a Brownian motion with drift, and jump component of finite variation. Jump diffusion can be studied on a microscopic scale by inelastic neutron scattering and by Mößbauer spectroscopy. Cybernetics 3 (63–69).T1 - Bayesian sequential testing for Lévy processes with diffusion and jump components Continuous Martingales and Brownian Motion. 2 2.1 General Quadrature Method For convenience, we provide a simple introduction to quadrature. All of the Matlab codes used within the study are available upon request. Optimal Stopping and Free-Boundary Problems. 4, we show the numerical results of different jump-diffusion models and put the method into practice. Sequential testing problems for Poisson processes. This technique attempts to replace one sequence of. Lecture Notes in Mathematics 1899, Springer (69–96). Simulation methods allow you to specify a popular variance reduction technique called antithetic sampling. A change-of-variable formula with local time on surfaces. A Bayes test of two hypotheses concerning the mean of a normal process (in Ukrainian). (Second Edition, First Edition 1977) Springer, Berlin. Probability Theory and Mathematical Statistics. On analitic methods in probability theory. On the optimality of the SPRT for processes with continuous time parameter. A framework for the optimal sparse-control of the probability density function of a jump-diffusion process is presented. Theory of Probability and its Applications 11 (541– 558). On Stefan’s problem and optimal stopping rules for Markov processes. Stochastics and Stochastics Reports 76 (59–75). The Wiener sequential testing problem with finite horizon. Stochastic differential equations and applications. The optimum choice of the instant for stopping a Markov process. Annals of Mathematical Statistics 24 (254–264). Sequential decision problems for processes with continuous time parameter. Stochastic Processes and their Applications 116 (1892– 1919). Sequential testing of simple hypotheses about compound Poisson processes. Sequential multi-hypothesis testing for compound Poisson processes. (French Edition 1978) North Holland, Amsterdam. Applications of Variational Inequalities in Stochastic Control. This research was partially supported by Deutsche Forschungsgemeinschaft through the SFB 649 Economic Risk. The authors thank the referee for a careful reading of the manuscript and useful comments. Stant, the sequential probability ratio test turns out to be optimal with constant boundaries which may only depend on the starting point of the observation process.
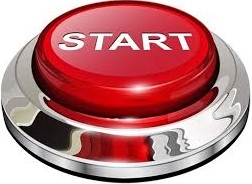